The world of protein design has always been one of the most exciting and challenging fields in science. From medicine to biotechnology, proteins are the building blocks that shape life itself. Understanding how proteins fold, function, and interact with one another has the potential to revolutionize medicine, disease treatment, and even how we tackle the world’s most pressing environmental issues.
For years, scientists have used various methods to predict and manipulate protein structures, but these techniques have often fallen short of creating proteins with novel or optimized functions. That’s where ESM3 (Evolutionary Scale Modeling 3) steps in. ESM3 is an advanced AI model developed to solve one of biology’s greatest challenges: predicting and designing proteins with unprecedented accuracy. This cutting-edge model merges protein sequence, structure, and function into a unified prediction system, pushing the boundaries of protein engineering.
In this article, we’ll explore what ESM3 is, how it works, and why it’s considered one of the most powerful tools for protein design in recent times. We’ll also dive into its applications, compare it with older models, and look at its future potential. By the end, you’ll understand why ESM3 is poised to become a game-changer in biotechnology and beyond.
What is ESM3?
Evolutionary Scale Modeling 3 (ESM3) is the latest iteration of a series of language models designed to predict protein structures and functions. Developed by EvolutionaryScale, ESM3 is a highly advanced AI model that uses deep learning techniques to unify three essential aspects of protein biology: sequence, structure, and function.
Protein design, historically a laborious and time-consuming process, involves predicting how a sequence of amino acids (the building blocks of proteins) folds into a three-dimensional structure. Once folded, the protein must perform a specific function, whether it’s catalyzing a reaction, binding to a target molecule, or performing a cellular task. The challenge has always been to predict these properties with high accuracy.
Traditional methods focused on one aspect of protein prediction—usually either sequence-to-structure or structure-to-function relationships. ESM3, however, takes a more holistic approach by simultaneously addressing all three domains: sequence, structure, and function. This is achieved through advanced AI techniques and large-scale evolutionary data, allowing the model to learn how proteins evolve, fold, and perform biological functions.
The innovation of ESM3 lies in its ability to generate novel proteins with tailored functions, something that was previously limited to random mutation-based trial and error. Now, with ESM3, scientists can generate proteins with precise structural and functional characteristics, opening up a world of possibilities for drug design, synthetic biology, and environmental solutions.
How ESM3 Works: The Technology Behind the Magic
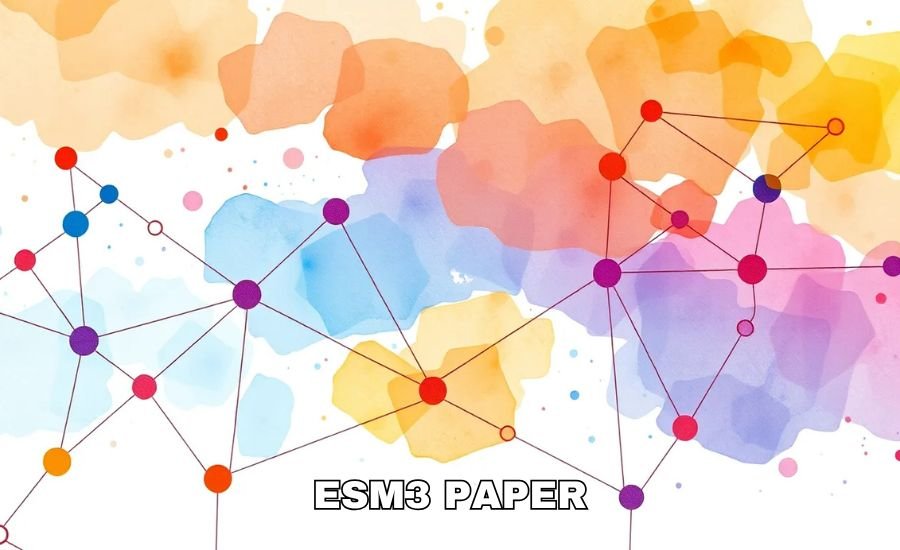
At the heart of ESM3’s power is its ability to process vast amounts of evolutionary data. The model has been trained on billions of proteins found in diverse environments—from the Amazon rainforest to the deep oceans. By examining this natural diversity, ESM3 learns to recognize and predict which protein sequences are likely to fold into functional structures and how they will behave biologically.
The ESM3 architecture is based on three key tracks: sequence, structure, and function. Each of these tracks is processed separately before being combined into a unified representation of a protein. This allows ESM3 to consider all aspects of protein design simultaneously, rather than focusing on one individual domain at a time.
ESM3 also uses multiple sequence alignments (MSAs) as a critical input for its training. MSAs help the model recognize evolutionary patterns and conserved regions within protein sequences. These patterns are crucial for understanding protein function and stability. The model is trained on data derived from proteins found in nature, such as those from microbes, plants, and animals. This vast evolutionary data set helps ESM3 understand how proteins evolve over time and how mutations affect protein structure and function.
The model is built on a bidirectional transformer architecture, which allows it to process information both forward and backward in the protein sequence. This bi-directional processing enables ESM3 to fully understand the relationships between amino acids in a sequence and their impact on the overall protein structure.
Additionally, ESM3 uses a geometric attention layer that considers the spatial relationships between amino acids in the three-dimensional protein structure. This attention mechanism helps the model focus on regions of the protein that are physically close to one another, further improving its ability to predict how proteins fold and interact with other molecules.
Key Features of ESM3
ESM3 brings several key innovations that set it apart from previous protein design models. Let’s explore some of its standout features:
1. Unified Sequence, Structure, and Function Prediction
Most previous models focused on either sequence-to-structure or structure-to-function prediction. ESM3, however, can simultaneously predict all three aspects of a protein. This unification is achieved by combining sequence data, structural information, and functional annotations into a single model. This ability to work across all three domains makes ESM3 incredibly powerful for protein design.
2. Generative Capabilities
ESM3 is a generative model, meaning it can generate new protein sequences based on a given set of structural and functional constraints. This generative capability is a major step forward in the field of synthetic biology, as it allows researchers to create proteins with novel structures and functions that have never been seen before in nature.
3. Scalability
The more data and computational power a model has, the better it performs. ESM3 has been trained on one of the largest GPU clusters in the world, giving it access to immense computational resources. As a result, the model has been trained on billions of protein sequences, allowing it to learn a vast range of evolutionary patterns and generate highly accurate predictions.
4. Multi-Modal Training
ESM3 uses multi-modal training, meaning it learns from both structural data (like X-ray crystallography or cryo-EM) and sequence data (like genetic sequences). This multi-modal approach enables the model to bridge the gap between protein sequence and structure, offering a more complete picture of how proteins function in nature.
The Science Behind ESM3
At its core, ESM3 operates on the principle that proteins evolve over time through natural selection. Functionally important regions of a protein are conserved throughout evolutionary history, while non-essential regions mutate. ESM3 learns these patterns by being trained on a massive dataset of proteins from a variety of organisms.
The model also learns about protein folding, which is the process by which a linear chain of amino acids folds into a specific three-dimensional structure. This folding process is highly complex and involves intricate interactions between the amino acids. By studying the relationships between sequence, structure, and function, ESM3 learns to predict how any given protein will fold based on its amino acid sequence.
The model’s success lies in its ability to predict protein folding with high accuracy, which has long been a challenging problem in molecular biology. By predicting how proteins fold, scientists can design new proteins that perform specific functions, such as binding to a particular target molecule or catalyzing a specific biochemical reaction.
Applications of ESM3 in Real-World Scenarios
ESM3 is not just a theoretical breakthrough—it has the potential to solve real-world problems in several key areas:
1. Drug Discovery
The ability to predict and design proteins with specific functions opens up exciting possibilities for drug discovery. ESM3 can help researchers design proteins that bind to disease-causing molecules, such as viral proteins or cancer cell receptors, to neutralize them. By designing novel antibodies or therapeutic proteins, scientists can develop more effective treatments for a range of diseases.
2. Enzyme Engineering
Enzymes are proteins that catalyze biochemical reactions, and they play a crucial role in many industrial processes. ESM3 can be used to design enzymes with enhanced properties, such as greater stability or higher efficiency. This could have significant implications for industries like agriculture, energy, and manufacturing, where enzymes are used to optimize chemical processes.
3. Synthetic Biology
Synthetic biology aims to design new biological systems that do not exist in nature. ESM3’s ability to generate novel proteins with tailored functions makes it an invaluable tool for synthetic biologists. Researchers can use ESM3 to create proteins that perform specific tasks, such as breaking down pollutants, producing biofuels, or even acting as biosensors for detecting environmental contaminants.
4. Environmental Solutions
One of the most exciting applications of ESM3 is in the development of proteins that can address environmental issues. For example, ESM3 has been used to design proteins that degrade plastics, helping to combat the growing issue of plastic pollution. With its generative capabilities, ESM3 can create enzymes that break down complex materials, offering a potential solution to one of the planet’s most pressing environmental problems.
Read Also: Body-Rubs-Nashville
ESM3 vs. Previous Models: What Makes It Stand Out?
ESM3 is not the first protein design model to hit the market, but it is certainly one of the most advanced. Let’s compare ESM3 with older models like AlphaFold, ProGen2, and ESM2:
1. AlphaFold
AlphaFold is a landmark model in the field of protein folding, but it primarily focuses on sequence-to-structure predictions. While AlphaFold is incredibly accurate in predicting protein structures from amino acid sequences, it does not directly handle protein function predictions. ESM3, on the other hand, integrates all three domains—sequence, structure, and function—allowing for a more holistic approach to protein design.
2. ProGen2
ProGen2 is another powerful protein design model, but it is more specialized than ESM3. It can accept sequences with some functional annotations and return the same, but it doesn’t offer the same level of flexibility and control over protein design as ESM3. ESM3’s ability to handle multi-modal inputs and generate novel proteins with tailored functions gives it a significant edge in many applications.
3. ESM2
ESM2, the predecessor to ESM3, was a significant step forward in protein design. However, it was limited in terms of scalability and its ability to generate proteins with highly specific functions. ESM3 takes things to the next level with its larger scale, multi-modal training, and ability to generate proteins with unprecedented accuracy and functionality.
Limitations and Challenges of ESM3
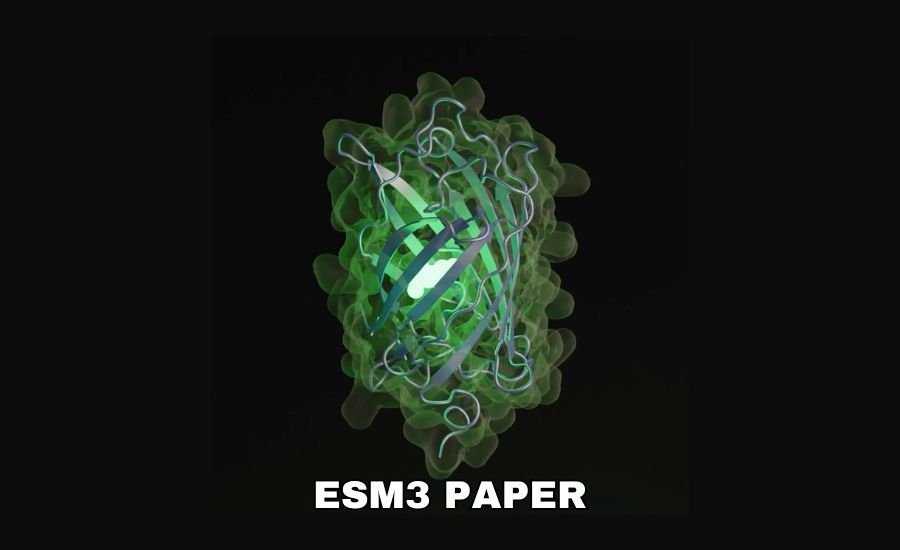
While ESM3 is a powerful tool, it is not without its limitations. One challenge is the model’s size and complexity. With 98 billion parameters, ESM3 requires massive computational resources to train and operate. This makes it difficult for many researchers to access and utilize the model effectively.
Additionally, ESM3 is still a relatively new model, and there may be areas for improvement in terms of accuracy and reliability. For example, while the model excels at generating novel proteins, its performance may not always match the level of specialized models in specific tasks, such as structure prediction.
Responsible Development and Ethical Considerations
As with any powerful technology, the development of ESM3 raises important ethical considerations. The ability to design and generate proteins with specific functions could have profound implications for biotechnology and synthetic biology. Researchers must ensure that these technologies are used responsibly, with careful consideration of potential risks and unintended consequences.
The Future of ESM3: What’s Next?
Looking ahead, ESM3 is poised to continue evolving. Future versions of the model may include more specialized versions for drug design, environmental applications, and even personalized medicine. As computational resources continue to grow and data sets expand, ESM3’s capabilities will only improve, bringing us closer to fully programmable biology.
Conclusion
ESM3 represents the cutting edge of protein design and engineering. By integrating sequence, structure, and function prediction, this generative AI model offers unprecedented control over protein design, with the potential to revolutionize fields like drug discovery, synthetic biology, and environmental solutions. While challenges remain, the future of ESM3 is bright, and it’s poised to play a pivotal role in the next generation of biotechnology.
ESM3 is more than just a tool—it’s a glimpse into the future of science, where artificial intelligence helps us understand and manipulate the very building blocks of life.
FAQs:
Q: What is ESM3?
A: ESM3 is a generative AI model that predicts and designs proteins by integrating sequence, structure, and function, making it a powerful tool for protein engineering and biotechnology.
Q: How does ESM3 work?
A: ESM3 uses deep learning and evolutionary data to learn how proteins fold, function, and interact, enabling it to generate novel proteins with tailored functions.
Q: What makes ESM3 different from AlphaFold?
A: Unlike AlphaFold, which focuses on predicting protein structures, ESM3 can predict and generate proteins with specific functions by considering sequence, structure, and function together.
Q: What are the main applications of ESM3?
A: ESM3 is used in drug discovery, enzyme engineering, synthetic biology, and environmental solutions like plastic degradation and disease treatment.
Q: What are the limitations of ESM3?
A: ESM3 requires large computational resources and may not always outperform specialized models in certain tasks, such as precise structure prediction.
Stay informed with the latest news and updates on: Techi Boomb!